Accelerating Queries with Reflections
A reflection is an optimized materialization of source data or a query, similar to a materialized view, that is derived from an existing table or view. A table or view that a reflection is derived from is that refection's anchor.
Dremio's query optimizer can accelerate a query against tables or views by using one or more reflections to partially or entirely satisfy that query, rather than processing the raw data in the underlying data source. Queries do not need to reference reflections directly. Instead, Dremio rewrites queries on the fly to use the reflections that satisfy them.
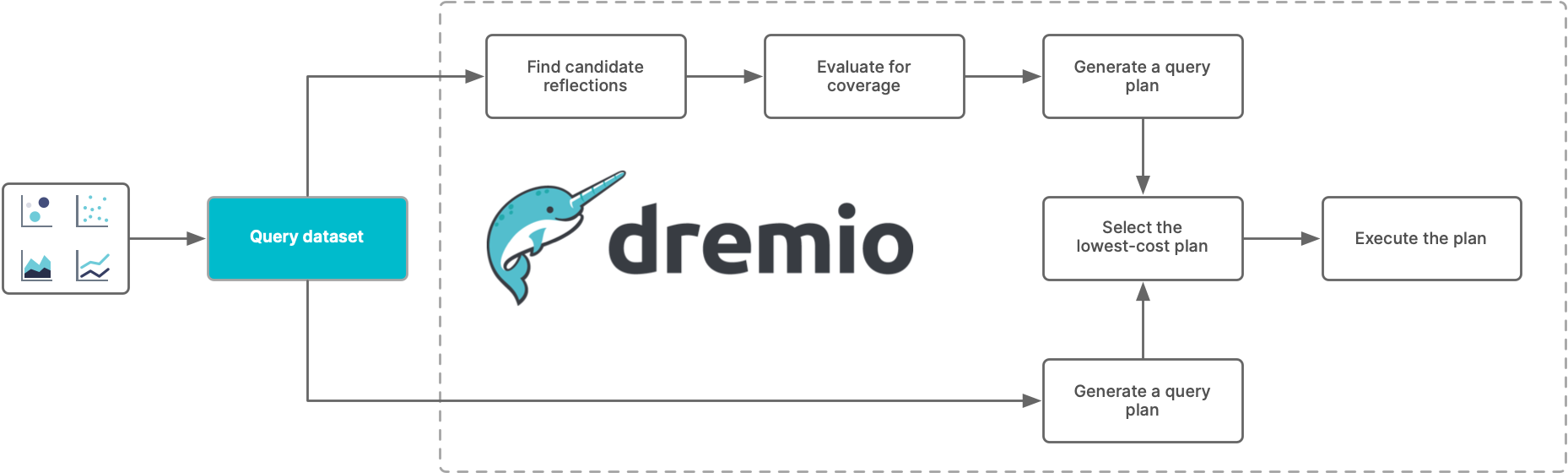
When Dremio receives a query, it determines first whether any reflections have at least one table in common with the tables and views that the query references. If any reflections do, Dremio evaluates them to determine whether they satisfy the query. Then, if any reflections do satisfy the query, Dremio generates a query plan that uses them.
Dremio then compares the cost of the plan to the cost of executing the query directly against the tables, and selects the plan with the lower cost. Finally, Dremio executes the selected query plan. Typically, plans that use one or more reflections are less expensive than plans that run against raw data. To get the best results from using reflections, see Best Practices for Creating Raw and Aggregation Reflections.
For example, suppose that three tables named customers
, orders
, and items
are created from data sources. A data engineer filling a requirement for allowing single queries across all three tables creates a view named order_detail
to join them:
SELECT *
FROM ((order INNER JOIN customer ON order.cust_id = customer.cust_id INNER JOIN item on order.item_id = item.item_id))
A business analyst wants to get a summary of the orders for each customer in the United States. She creates a view named customer_summary_US
that is defined by this query on order_detail
:
SELECT cust_name, city, COUNT(item_id), SUM(item_price) FROM order_detail
WHERE cust_country = 'US'
GROUP BY cust_name
ORDER BY SUM(item_price) DESC
Because this query is computationally intensive, takes a bit of time to run, and is a query that she runs frequently, the business analyst, together with the data engineer, creates a reflection from her view, following best practices. They give the reflection the same name as the view.
The relationships between the reflection, tables and views, and the data sources can be represented like this:
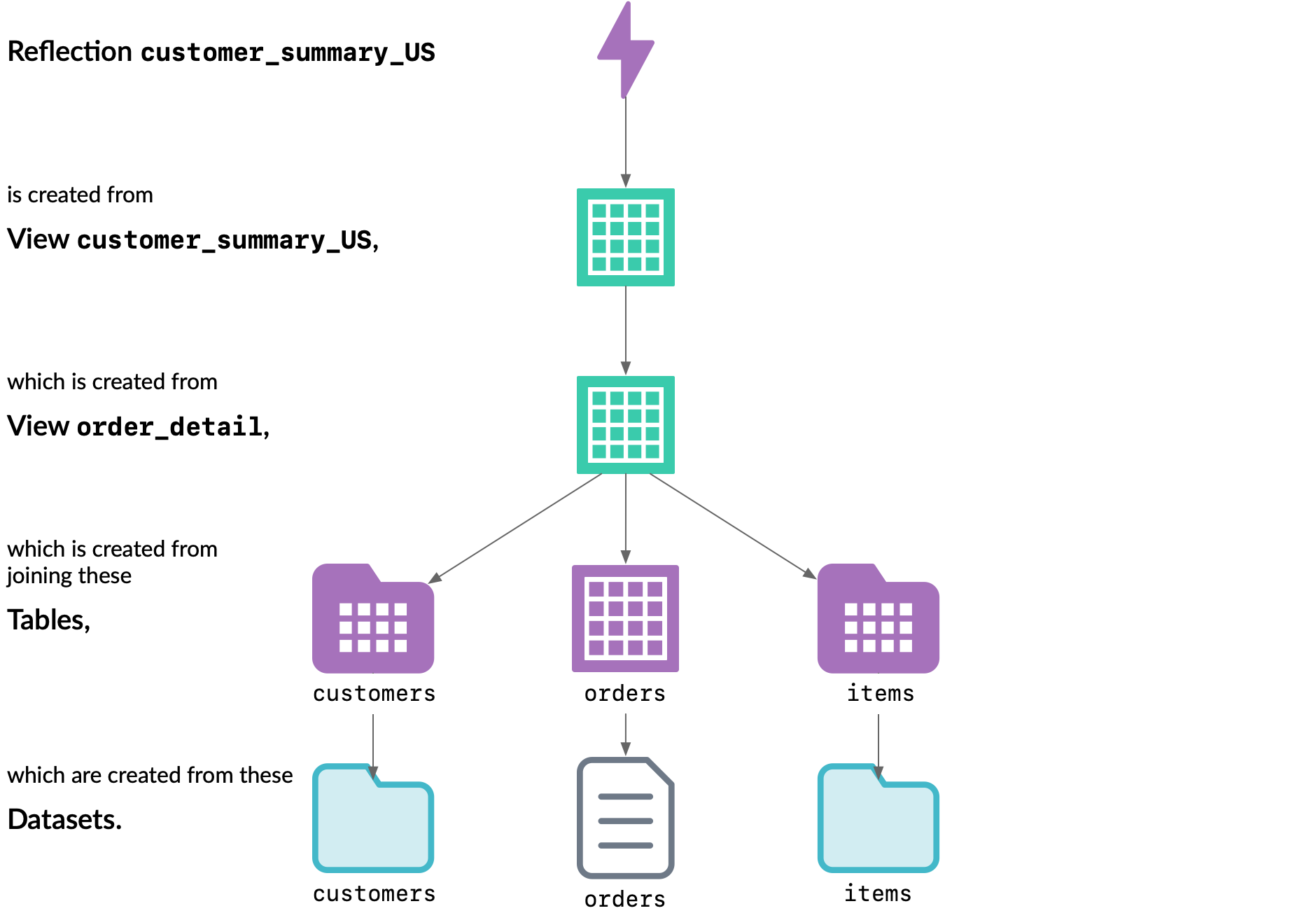
When the business analyst runs her query from her BI client application, Dremio's query planner runs the query against the reflection, not against the view customer_summary_US
, even though her query references the view. Her query runs much faster because the Dremio engine running it does not have to descend through the tables and views to the raw data in the data source.
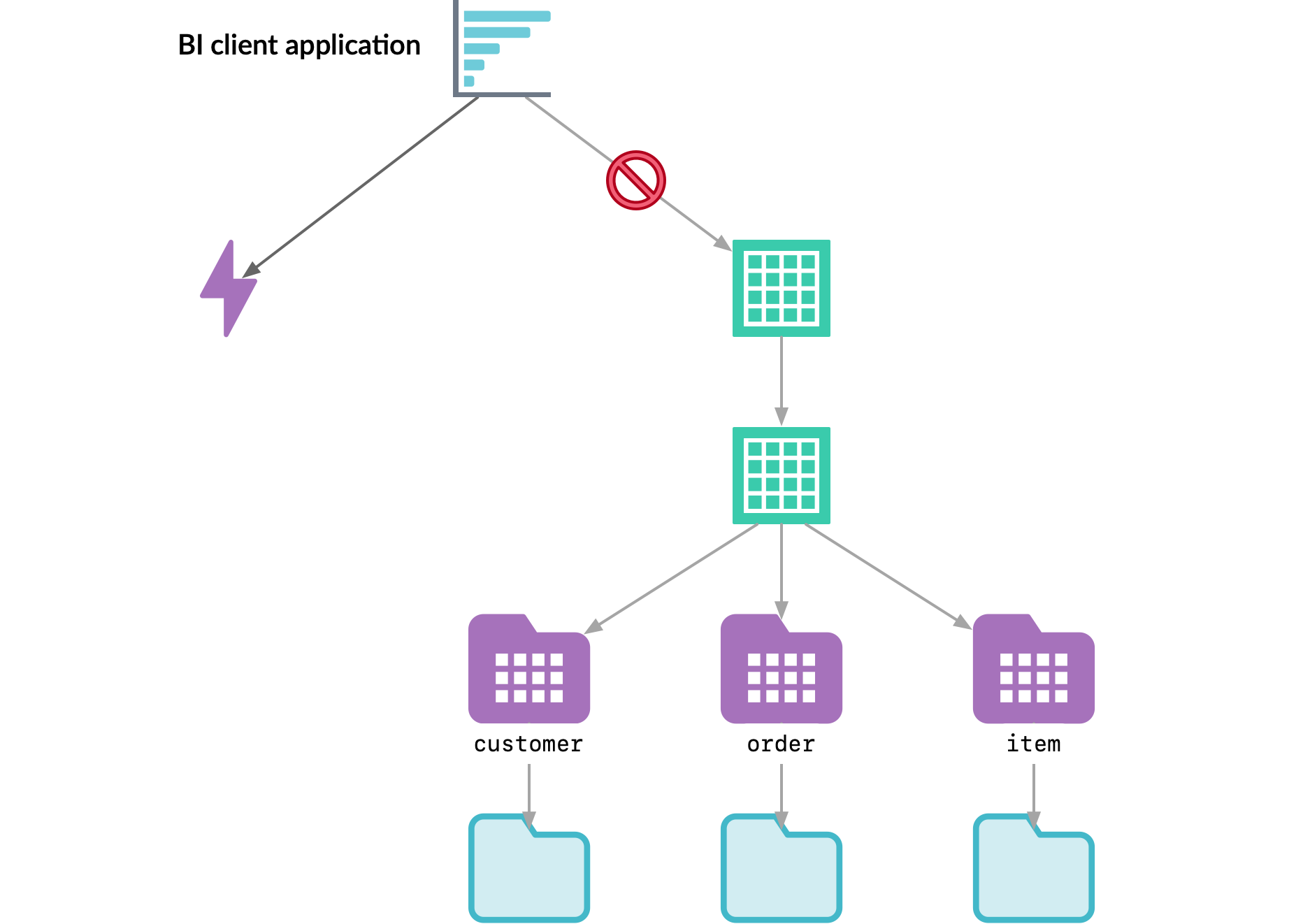